AI Explainability: What That Means and Why it Matters in Healthcare
Is AI a topic we should be focused on in the medical device industry?
Artificial Intelligence has taken great strides and is a very topical issue now for medical device manufacturers.
Xavier Health works to bring the industry and the FDA together in a collaborative setting. They break down barriers, generate ideas and explore what-ifs. Their conferences are very purpose-driven and aim to advance the industries for the betterment of patient health. I have been to these conferences myself, and encourage you to check them out. The level of collaboration between the FDA and participants is impressive and very useful.
Here’s what Marla and I chatted about:
Marla recently attended a panel on Capitol Hill, focused on AI. She met with Congressmen Delaney, Matsui and Bucshon, in a summit coordinated by IBM. This was put together as there has been a lot of concern about AI and the implications for the end-user (in this case, the healthcare provider).
The goal of the panel was to address explainability, what this means, and why it is important to the end-user. If you think of a healthcare provider and the use of AI in diagnoses - how can they have confidence in the outcome they’re being shown?
Transparency is a part of explainability. It links the credibility of the inputs to the outputs. This helps to give users more confidence in the outcome. The aim is to build this explainability so that the healthcare provider can have confidence when they’re standing in front of a patient.
AI gives us the opportunity to advance in healthcare beyond where we are today. The panel recognized the importance of collaboration, with the industry and government coming together to give healthcare providers the tools they need.
Why the industry and FDA are coming together
Why are the industry and the FDA coming together to advance AI in healthcare? AI is certainly a hot topic everywhere we go, but why does it apply to healthcare?
For the last 20-30 years we’ve exploded the amount of data we’re collecting, however, it largely sits unused on the shelf. We recognize that there’s a tremendous amount of power in that unused data, and that you can use AI to analyze it and reach correlations that we previously didn’t realize existed.
Basically, the FDA and the industry are now recognizing that there’s a lot they could be doing with all of this data, and have come together with a passion for utilizing it for better patient outcomes. AI gives us the opportunity to be more proactive in medical device design and development, allowing for better service to the end user.
Use of AI goes from being proactive to predictive, then from predictive to prescriptive. This means that if I can predict a failure will occur when certain conditions are met, then I can prescribe the controls and conditions. I can prescribe all the different variables in the materials themselves and the manufacturing conditions so that I avoid the occurrence of the failure conditions.
There are teams looking at “continuous product quality assurance.” This means asking “what is all the data that you have in your hands?” This might include numbers or even data in Word documents using natural language. It’s about taking all of this data into account. For example, AI can analyze the high and low ends of your control ranges during manufacturing, then come up with the conditions that always lead to failure.
AI also has the potential to play a key role in auditing. When a human auditor comes in, they can only touch a fraction of the data that a company has produced over the last couple of years. However, AI can be scanning data all the time. It could identify correlations we never realized existed. For example, what if weather came into how the product or an ingredient in the product behaved?
This application of AI is a good example of why platforms like Greenlight Guru exist for eQMS. It is designed to be a “single source of truth” which makes it simple for AI programs to pull data and analyze it.
How the industry and FDA are working through Xavier University
One of the questions they are working on is; where is all of this data? Data scientists will often refer to a “data lake” - you have to get data into a common place so that the AI can work on it. Data might include everything from weather to product specifications and there are different break-out groups being set up to work on these from the next AI Summit.
Phase two of the work is looking at assessing open FDA data to scan it and look for correlations among the different data points.
They are also looking at what needs to be done to clean up data so that it is more useful. For example, if there are several incidents of a recall reported through a law firm that only say “there was an issue,” that data is not very useful in terms of being able to understand what was behind the issue. AI can be used to weed out the garbage data and focus in on what’s meaningful. It can start to look across recalls and reports across different sites to identify any correlations.
How do you evaluate and validate a system that is continuously working and learning? They have a team working on this question too. Explainability comes into this too - being able to explain how it reached its outcomes and the credibility of the inputs.
That piece around how to train an algorithm is one of the major challenges they are working on. It’s a huge paradigm shift from previous procedures.
Challenges for AI in healthcare
There are a number of challenges which are being worked on in the industry. For example, just knowing where the data is and that it is accurate is one. Only around three to four percent of adverse events are actually reported to the FDA, which leaves a large amount of untapped data.
Another challenge is demonstrating the credibility of AI outputs if we’re not using validation. It’s not just the FDA, it’s global regulation that needs to consider this. Will changes be needed to support this new technology?
Some companies are still using paper-based systems - this excludes them from the big data world. Their first step is that their data needs to be in an electronic format to be able to access the benefits of AI. Honestly, in today’s world, if you’re still on paper-based systems, I believe you’ll be left behind.
Software as a medical device is another consideration. When you look at software, there are always iterations and updates. You can’t have a six-month approval process for the FDA to look at it; otherwise, it quickly becomes obsolete. There is a working group looking at how the FDA can give approval on a real-time basis.
How AI can transform our operations
AI can be a key part of the product in the medical device industry. Devices can be “smart” and identify correlations that are patient-specific, providing more personalized service.
Feedback for post-market surveillance is another key application of AI. Companies can stay on top of what is happening and get to that “prescriptive” stage mentioned earlier.
For some people, there is still fear associated with AI. This is understandable if you read a lot of the publications put out about it! The bottom line is to understand the real context behind any story. For example, if it’s a story talking about how the AI failed, take a look at the failure rate behind it. Perhaps the AI is 97% accurate in a case where humans average 82% accuracy.
The other thing is to understand how it works. The idea is to use it to augment human decisions, not make decisions for us. It gives us the opportunity to look at all the data we can’t otherwise look at, to make as informed a decision as we can. Sometimes you will be wrong, but with AI we can do better than we do without it.
Check out the programs available at Xavier Health, including the next AI Summit.
Jon Speer is a medical device expert with over 20 years of industry experience. Jon knows the best medical device companies in the world use quality as an accelerator. That's why he created Greenlight Guru to help companies move beyond compliance to True Quality.
Related Posts
What You Need to Know About the 510(k) Submission Process
A Review of the Most Important Medical Device Industry Topics in 2016
Why Usability Matters
Get your free resource
6 Ways AI is Transforming Healthcare
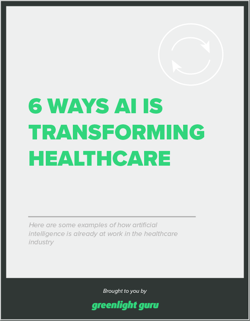